Abstract
Artificial intelligence (AI) algorithms have an enormous potential to impact the field of radiology and diagnostic imaging, especially the field of cancer imaging. There have been efforts to use AI models to differentiate between benign and malignant breast lesions. However, most studies have been single-center studies without external validation. The present study examines the diagnostic efficacy of machine-learning algorithms in differentiating benign and malignant breast lesions using ultrasound images. Ultrasound images of 1259 solid non-cystic lesions from 3 different centers in 3 countries (Malaysia, Turkey, and Iran) were used for the machine-learning study. A total of 242 radiomics features were extracted from each breast lesion, and the robust features were considered for models’ development. Three machine-learning algorithms were used to carry out the classification task, namely, gradient boosting (XGBoost), random forest, and support vector machine. Sensitivity, specificity, accuracy, and area under the ROC curve (AUC) were determined to evaluate the models. Thirty-three robust features differed significantly between the two groups from all of the features. XGBoost, based on these robust features, showed the most favorable profile for all cohorts, as it achieved a sensitivity of 90.3%, specificity of 86.7%, the accuracy of 88.4%, and AUC of 0.890. The present study results show that incorporating selected robust radiomics features into well-curated machine-learning algorithms can generate high sensitivity, specificity, and accuracy in differentiating benign and malignant breast lesions. Furthermore, our results show that this optimal performance is preserved even in external validation datasets.
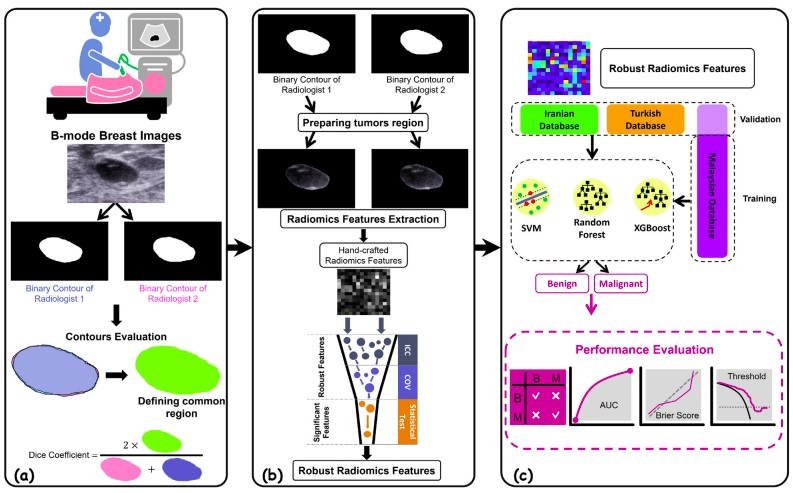