Objectives
The purpose of this study was to evaluate a computer-aided diagnostic system with texture analysis to improve radiologists’ accuracy in the identification of breast tumors as malignant or benign.
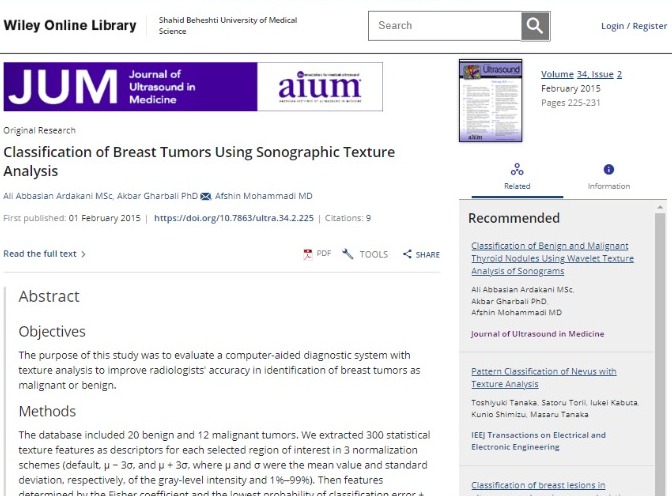
Methods
The database included 20 benign and 12 malignant tumors. We extracted 300 statistical texture features as descriptors for each selected region of interest in 3 normalization schemes (default, μ − 3σ, and μ + 3σ, where μ and σ were the mean value and standard deviation, respectively, of the gray-level intensity and 1%–99%). Then features determined by the Fisher coefficient and the lowest probability of classification error + average correlation coefficient yielded the ten best and most effective features. We analyzed these features under two standardization states (standard and nonstandard). For texture analysis of the breast tumors, we applied principal components, linear discriminant, and nonlinear discriminant analyses. First–nearest neighbor classification was performed for the features resulting from the principle component and linear discriminant analyses. Nonlinear discriminant analysis features were classified by an artificial neural network. Receiver operating characteristic curve analysis was used to examine the performance of the texture analysis methods.
Results
Standard feature parameters extracted by the Fisher coefficient under the default and 3σ normalization schemes via nonlinear discriminant analysis showed high perform-ancefor discrimination between benign and malignant tumors, with sensitivity of 94.28%, specificity of 100%, accuracy of 97.80%, and an area under the receiver operating characteristic curve of 0.9714.
Conclusions
Texture analysis is a reliable method and has the potential to be used effectively for classification of benign and malignant tumors on breast sonography.