Abstract
Background:
Early detection and reliable diagnosis of breast cancer could lead to improved cure rates and reduce mortality and management costs.
Objectives:
To explore the potential of texture analysis based on run-length matrix features for classifying benign and malignant breast tumors in ultrasound imaging.
Methods:
A total of 70 breast tumors (38 benign and 32 malignant) have used in the proposed computer-aided diagnosis system. Twenty run-length matrix features have extracted for texture analysis in three normalizations (default, 3sigma, and 1% – 99%). Linear discriminant analysis and principal component analysis have employed to transform raw data to lower-dimensional spaces and increase discriminative power. The features have classified by the first nearest neighbor classifier.
Results:
The features under 3sigma normalization have designed via Linear discriminant analysis indicated high performance in classifying benign and malignant breast tumors with a sensitivity of 96.87%, specificity of 100%, accuracy of 98.57%, positive predictive value of 100%, and negative predictive value of 97.43%. The area under receiver operating characteristic curve was 0.992.
Conclusions:
Run-length matrix features had a high potential to characterize and could help radiologist to diagnosis breast tumors.
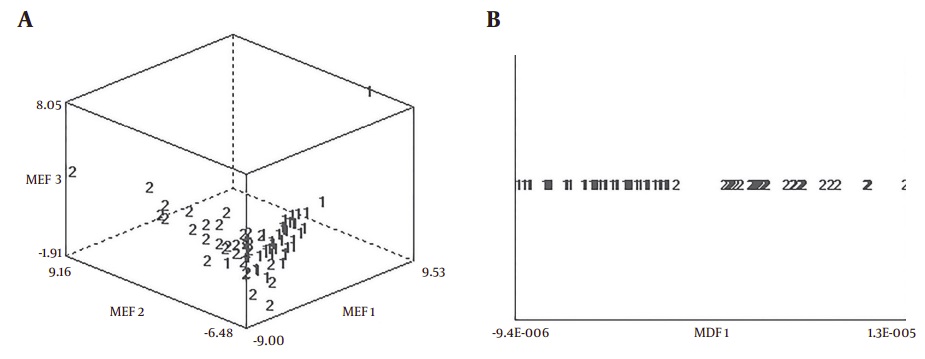
Benign and Malignant Breast Tumors Respectively