Abstract
Objectives
Deep learning algorithms have shown potential in streamlining difficult clinical decisions. In the present study, we report the diagnostic profile of a deep learning model in differentiating malignant and benign lymph nodes in patients with papillary thyroid cancer.
Methods
An in-house deep learning-based model called “ClymphNet” was developed and tested using two datasets containing ultrasound images of 195 malignant and 178 benign lymph nodes. An expert radiologist also viewed these ultrasound images and extracted qualitative imaging features used in routine clinical practice. These signs were used to train three different machine learning algorithms. Then the deep learning model was compared with the machine learning models on internal and external validation datasets containing 22 and 82 malignant and 20 and 76 benign lymph nodes, respectively.
Results
Among the three machine learning algorithms, the support vector machine model (SVM) outperformed the best, reaching a sensitivity of 91.35%, specificity of 88.54%, accuracy of 90.00%, and an area under the curve (AUC) of 0.925 in all cohorts. The ClymphNet performed better than the SVM protocol in internal and external validation, achieving a sensitivity of 93.27%, specificity of 92.71%, and an accuracy of 93.00%, and an AUC of 0.948 in all cohorts.
Conclusion
A deep learning model trained with ultrasound images outperformed three conventional machine learning algorithms fed with qualitative imaging features interpreted by radiologists. Our study provides evidence regarding the utility of ClymphNet in the early and accurate differentiation of benign and malignant lymphadenopathy.
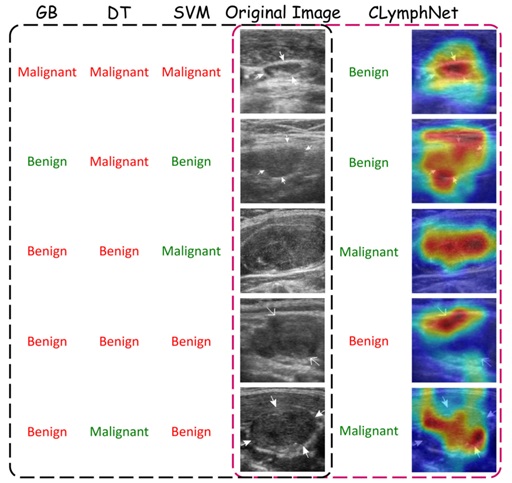
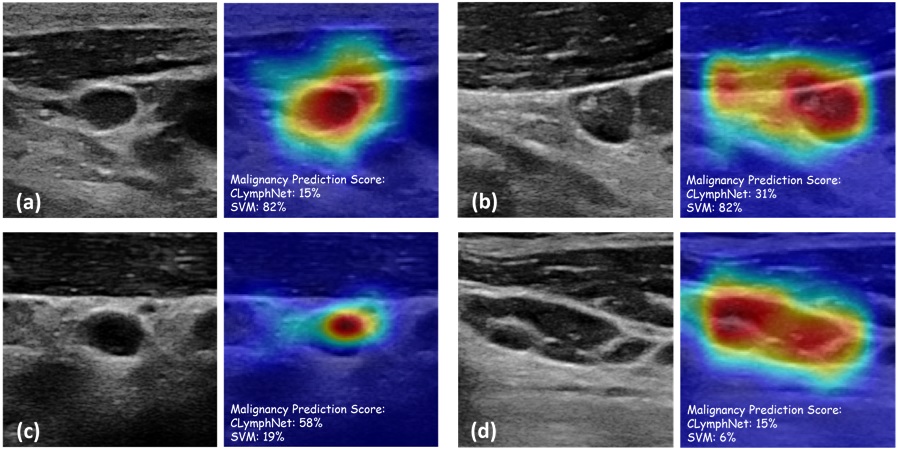