Abstract
Aims:
The aim of this study was to evaluate texture analysis (TA) in pre-contrast injection MR images to improve accuracy and to identify subtle differences between enhancing lesions (ELs), non-enhancing lesions (NELs) and persistent black holes (PBHs).
Materials and methodology:
The MR image database comprised 90 patients; 30 of whom had only PBHs, 25 had only ELs and 35 neither EL or PBH. These were assessed by the proposed TA method. Up to 300 statistical texture features were extracted as descriptors for each ROI/ lesion. Differences between the lesion groups were analyzed and evaluations were made for area under the receiver operating characteristic curve (Az) for each significant texture feature. Linear discriminant analysis (LDA) was employed to analyze significant features and increase power of discrimination.
Results:
At least 14 texture features showed significant difference between NELs and ELs, NELs and PBHs, and ELs and PBHs. By using all significant features, LDA indicated a promising level of performance for classification of NELs and PBHs with Az value of 0.975 that corresponds to sensitivity of 94.3%, specificity of 96.3%, accuracy of 95.5%. In classification of ELs and NELs (or PBH), LDA demonstrated discrimination performance with sensitivity, specificity and accuracy of 100% and Az of 1.
Conclusions:
TA was determined as a reliable method, with potential for characterization and the method can be applied by physicians to differentiate NELs, ELs and PBH in pre-contrast injection MRI imaging.
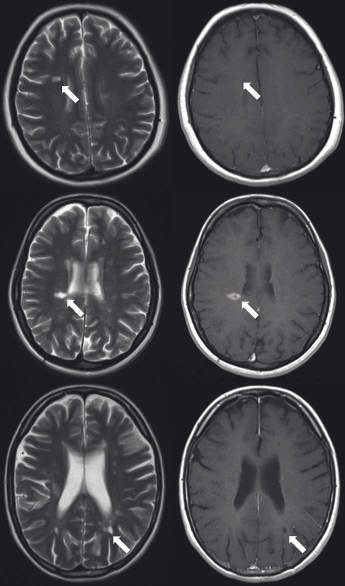